Mechanistic Modeling and its Role In Drug Discovery
What is mechanistic modeling, and why is it important for drug discovery?
Before we discuss mechanistic modeling, it is important to understand the definition of empirical pharmacokinetics pharmacodynamics (PK PD). This refers to studying the drug’s behavior in a body, how it is cleared, and what it does to your body, by using data obtained in preclinical testing, for example in a mouse, and fitting it to a curve to make a prediction on what doses to use on humans. This dose must be both safe and effective in humans. Translation of this data from preclinical to clinical is difficult and allometric scaling is not comprehensive. The biological processes in humans compared to other species are not just a function of height, sex, weight, surface area, and other such parameters. The mechanism matters – parameters such as the turnover rates of cells, numbers of cells, and number of sites per cell in a population of patients with a certain indication in which there are distributions of, for example, ligands or cells. Even within a similar biological mechanism of action, those ranges are different and the properties of your drugs or medicines matter on what indication you go through. With typical PK PD, we take our most recent dataset and make a forward prediction. However, when we have the mechanism, we can begin this process much earlier and be more forward-looking. With mechanistic modeling, we include factors like the drug's on and off rates, and we aim to understand in vitro potency or function. This helps us create a more mechanistic and accurate model of how a therapeutic will work in humans. We can essentially make better predictions because we incorporate more data and test more hypotheses, improving efficacy and safety in humans further down the pipeline. Ultimately this helps the drug development process by predicting what’s best in class, which experiments to prioritize before phase 1, what the variables are and how they can impact the safe starting dose, how much drug is efficacious, and how much is toxic. The ability to use modeling to make such predictions can accelerate candidate selection and support clinical trial design.
[Poster] Quantitative systems pharmacology (QSP) model for preclinical to clinical translation of antibody-drug conjugates (ADCs) |
How does mechanistic modeling differ from traditional modeling?
With traditional PK/PD modeling, you may take the most recent set of data (for example, an in vitro mouse model) and you would do parameter estimation to fit using arbitrary parameters and doing curve fitting. Going from preclinical to clinical predictions, you might, for example, compare the size of the mouse used and the PK in the mouse, to an experiment run in a dog and how big is the dog relative to the mouse. After that, you may go into a non-human primate and compare the sizes to the other two species. In an attempt to understand a safe starting dose in humans, you may extrapolate from the data gathered.
With mechanistic PK/PD approaches, we consider the target binding kinetics (kon and koff), or the affinity of the drug to the target it is intended to bind to. Assuming the example of a biologic, we would consider the mechanism of action of the therapeutic and to maybe first understand in vitro potency or function, we would set up the differential equations to match that in vitro data set as closely as possible to the real experimental system. This would consider the number of cells, sites per cell, if you are pre-incubating with a ligand or not, if the systems are being activated, and then at the right time point, you are putting in the drug. Following that perhaps you look at a readout of function, whether that is cytokine release, proliferation, or death. In humans, such parameters are different and there are additional considerations such as feedback loops, autocrine and paracrine signaling, differences in target expressions, and more. With mechanistic modeling, you can model all of these things to help better translate from the in vitro system into the human system.
When we translate from that preclinical to clinical space we have more certainty in our predictions than in traditional approaches. We mechanistically not only understand the risks of the drug but also the relevant biology and we have a better understanding of the uncertainty and variability before we go into humans. We can also incorporate published competitor, preclinical, and even clinical data to help strengthen our predictions. Traditional modeling does not do this. With mechanistic models through uncertainty analysis and variability, we can start translating forwards and backward to consider what’s best in class, the most important experiments, the most critical unknowns, and how variable a clinical trial could be.
“When we translate from that preclinical to clinical space we have more certainty in our predictions as opposed to traditional approaches.”
Where in the pipeline does Applied BioMath get involved, and when should mechanistic modeling be brought in?
Applied BioMath can help at nearly every stage of the pipeline, from the target identification stage to the late-stage clinical phase. Smaller biotechs often approach us when they are preparing for their IND and need accurate human dose predictions. For larger companies, we can come in at the project start to provide early insights. Ideally, mechanistic modeling should be part of the process right from the beginning to guide lead optimization and experiment prioritization, and potentially produce a better molecule.
“I would argue the best time to work with [Applied BioMath] is right when that project starts because oftentimes we’ll find [...] for example, with a bispecific immune cell, tumor cell engager - maybe their therapeutic index is too low. Maybe their molecules are too potent [...] and they could have had a better molecule.”
When our partners have multiple drug concepts of interest, they will also come to Applied BioMath to do the first round of assessment at the target stage, build the right model with the appropriate level of complexity, to help determine which would be ideal candidates for pursual. We can further support in mathematically prioritizing experiments to do next by reviewing parameters that may impact the uncertainty and variability of human dose predictions down the line. As the project progresses, we can start factoring in relevant downstream biology and determine which assays will help make better predictions. One can even use modeling to understand which in vitro experiments to use and how to best design them, effectively using them for lead identification or optimization. Mouse models can be incorporated to constrain parameters better and make stronger predictions. Modeling doesn’t just help with candidate selection but also past the IND stages where our partners will be interested in population pharmacodynamics (PopPK) informing with real, clinical data to understand uncertainty and variability to design phase 1b, phase 2, or even launch steps. Model-informed drug development (MIDD) has a role in every step of the drug development journey.
“[...] through the whole spectrum [...] anyone who used mechanistic modeling, has a competitive advantage. It helps you really think and test hypotheses and generate hypotheses much more quickly [...]”
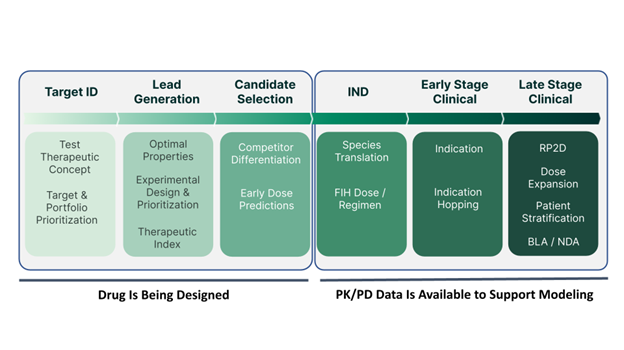
How much data do groups need to have at this stage for you to be able to build the model?
What’s key is not necessarily more data but rather the right data. Typically the amount of data required is the same as the data that teams would generate otherwise. Applied BioMath may suggest alternative experiment designs to achieve this. When we incorporate parameters like the number of cells, sites per cell, concentrations, etc., the overall predictions are stronger. Much of this data can often be obtained from the public domain. For certain parameters, you may find that the uncertainty in the predicted PK PD is unaffected, in which case, it may be justified as a parameter of less importance. Similarly, there may be other parameters like protein synthesis where subtle changes can give you drastically different outputs in your predicted human PK PD. Essentially, even very early on, this informs potentially critical experiments to run because it might impact go/no-go decisions for drug developers and may impact how much you have to perturb the system.
“It’s not that much more [data…]. It’s just thinking about your data collection differently.”
Try out a 2-week trial with predefined models or request a demo today
What is the process like for groups as their models are being developed?
Applied BioMath works very closely with our partners to build the right model in the right timeframe. The first part involves understanding the indication, how a partner’s drug works, and the data they have generated. We consider what data they plan on generating and when that may become ready. We are cognizant of their timelines as well throughout this process, whether it’s in preparation for an IND or for an upcoming scientific advisory board meeting. Next, we discuss which parts of the project we have already worked on and begin building the model diagram of what we will develop mathematically. We consider the data that are important in building the model and test the model predictions. We continue to fine-tune the parameter estimation, incorporating the in vitro and/or ex vivo data, adding in relevant biology, and following the same iterations through species. Our partners are present throughout this whole process seeing what assumptions are going into the models, what parameters are being used, and how the model development is coming along. Together we discuss what goes into the model to make it better until we are ready to make those first-in-human dose predictions. At the penultimate milestone, we make those human dose predictions at which point there may be questions and another round of assessments. Finally, we will write the model development process summary in a report so our partners can make references to that in their future reports, for example in their IND. Generally speaking, this is how the process would look for the team we work with and it would be modified based on what questions our partners are trying to answer.
[Article] Transforming Preclinical Research with Mechanistic PK/PD Modeling Approaches |
The FDA has endorsed MIDD - what does this mean for the integration of modeling into R&D?
The FDA's endorsement of Model-Informed Drug Discovery and Development (MIDD) reflects their increasing expectation for better mechanistic dose predictions. Companies that embrace mechanistic modeling are more likely to have a competitive advantage when dealing with the FDA. Mechanistic modeling can lead to better ROI, reduced late-stage attrition rates, and ultimately, lower drug prices while maintaining high-quality research.
How does mechanistic modeling apply to cell and gene therapies?
Cell and gene therapies pose even more complex questions, such as dosing cells, changes in phenotype, and the impact on patient outcomes. In essence, the same questions are being considered but they are even more difficult. For example, if you have a cell therapy, how would you define “dose?” What do you mean by PK? How do you think about steady state? How do you think about changing phenotype? How do you think about PD? Are you counting on your newly engineered cells to change cytokine synthesis? How do those cytokine differences impact the balance of regulatory T cells and effector T cells? This could impact tumor-infiltrating lymphocytes (TILs), which could impact tumor growth inhibitors or direct cell killing in sickle cell disease for example, where you are thinking about how your engineered platelet might help patients who are suffering.
Because the questions are even more difficult, mechanistic modeling becomes even more crucial for these therapies to help navigate the combinatorial complexity and cost while ensuring a successful patient outcome.
“[T]he combinatorial complexity and cost in doing all of the experiments that you might have to do or the dosing that would have to happen in humans, it just gets more and more expensive.”
A more detailed discussion of mechanistic modeling and how it helps refine and accelerate the drug development process can be heard on the podcast.